Click here for a very-high level summary
Robotics became very consensus very quickly. This happened because VCs are more aggressively extrapolating trend lines than ever before, and the concept of grasping Scaling Laws supercharged this for all AI adjacencies. This says something about how founders and investors should think about building & funding technology companies long-term, because consensus happens so quickly and aggressively, there are a ton of low-conviction dollars in the market, and competition means it’s harder to build durable and compounding moats. Ok now read the actual post and don’t be lazy.
A fairly profitable investment strategy in technology has always been understanding second-order effects of certain first-order inflection points. Put simplistically, IF X is probably happening, then Y could happen and thus if we believe the likelihood or scale of Y happening is higher than the broader market believes, we should speculate on Y.
A thinking of technology progression, derivative understanding, and imagination, generally.
But something has happened in the past few years that has potentially compressed the returns in this strategy and also has shifted dynamics of how these next-order effects and resulting emerging categories get capitalized and how companies can build advantages.
The lens of AI and the rise of attention and consensus within Robotics specifically is a good lens in which to view this change through.
The Fallacy of Seeing The Present Clearly & Alpha of predicting the future
There’s a recent Lesswrong post that looked at the concept of higher order forecasts and broke them down from ground truth all the way to Third-Order Forecasting which gives a decent framework for how to think about parsing changes in technology markets and startup investing.
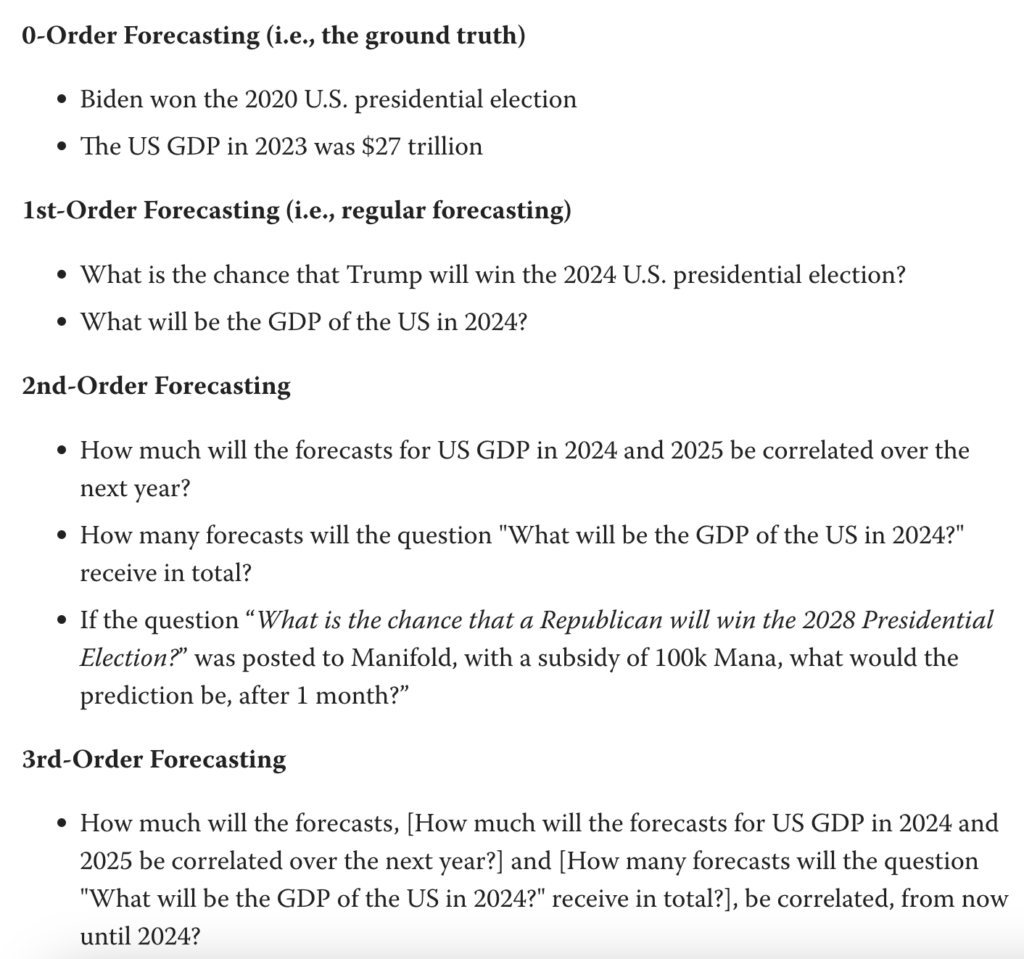
For my entire career, I have largely tried to think about how to build an investment firm surrounding the idea of identifying and speculating on future inflection points and using these learnings to then capture value from having a nuanced and precise view on the cascading effects that result from the change. We (Compound) build out a collection of futures we believe in and use capital and time to help brilliant people accelerate and/or materialize those forecasted futures.
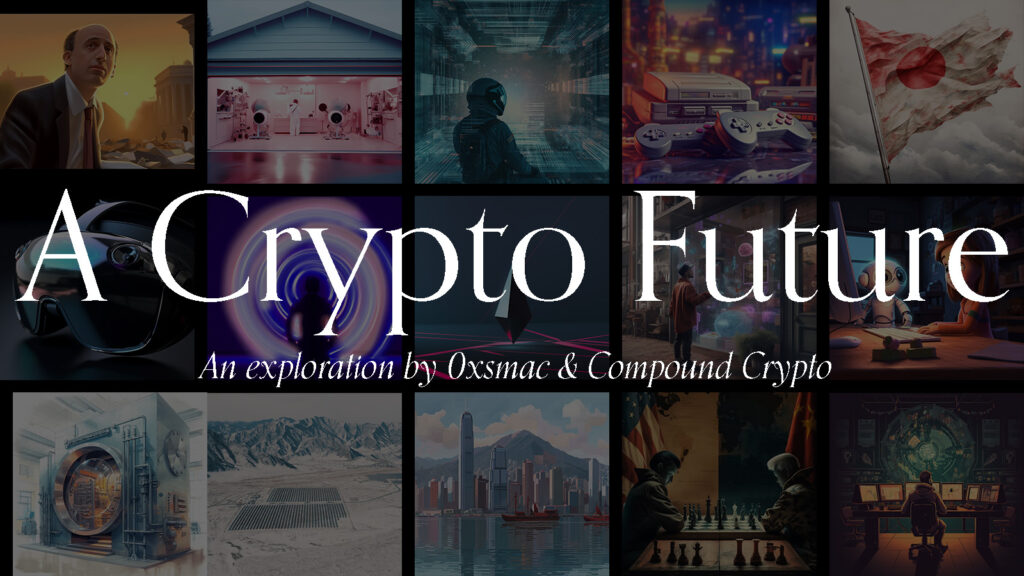
We’ve built many frameworks and processes internally around forecasting both at a higher level of technology development as well as more granular levels of industry and societal development. This has, at times, led to public-facing research such as A Crypto Future, in which we reasoned year-by-year about how the crypto would change as an industry and how the world would change around crypto.
Despite my conviction in this approach, there have always been screams from many other VCs that predicting the future was incorrect, with many pointing to the most bastardized quote in all of venture, said by the legend Matt Cohler: “Our job as investors is to see the present very clearly, not to predict the future.”
I have loudly debated the nuance of this statement as an alpha generating strategy at the early stage and have only gained conviction in this stance in a world in which there are more than a few venture firms with over $100M who all know each other and collaborate (like the world used to be).
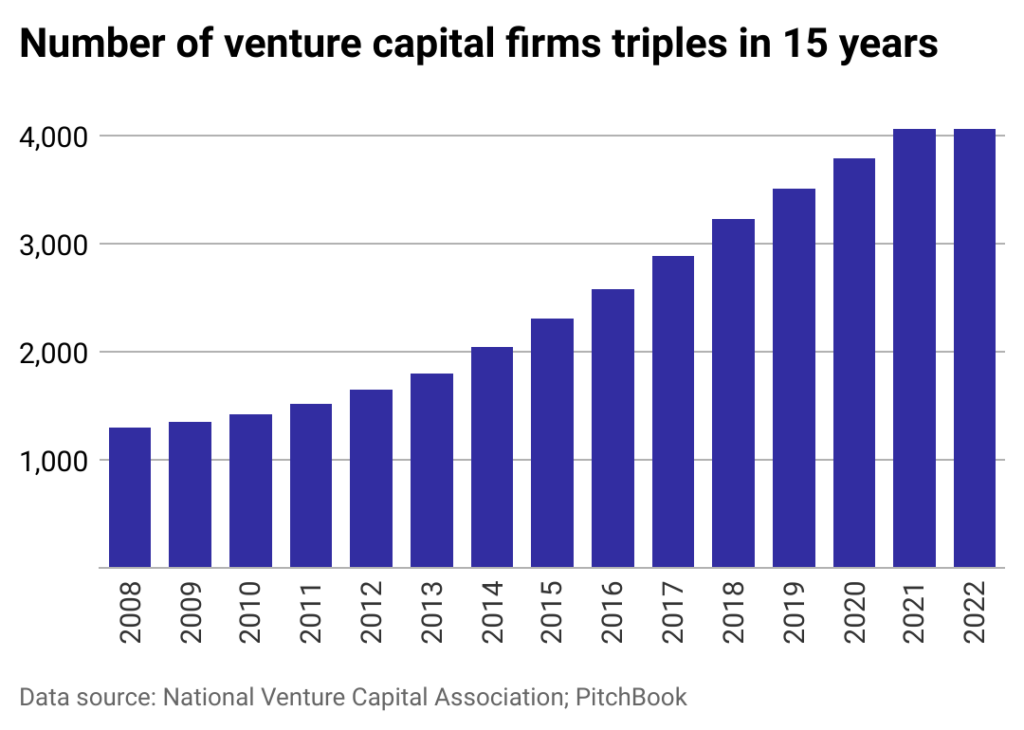
I would argue to see the present clearly today means to pay 75-200x+ ARR ahead of any durable product-market fit on a term sheet that sits alongside 4-6 others, or it means you will sneak in a small check that won’t meaningfully impact fund performance. This may work for some in building a single beta-catching fund, but I don’t believe one can build a consistently alpha-generating firm in this dynamic anymore.1This is a point for another post about how growth funds may be actually the most defensible mechanism within venture today because of this exact dynamic as post-PMF and growth is where you should be best at seeing the present clearly and in an outsized way.
Because of this return-compressing dynamic, a large number of investors spend their lives frantically looking to understand what is slightly next while abandoning what feels slightly over. This is partially because the forecasting of simplistic adjacencies is easy to rally a partnership around, easy to sell LPs on, and easy to gain conviction on quickly because you feel a bit smarter than simplistic first-order thinkers who just bet on the perceived leaders in a given space.
However, as this post is focused on the early-stage, what this often means is that in order for this to be the right strategy, the early perceived leaders must persist. This is an open question in highly competitive and crowded (consensus) areas where because of the scale of capital in private markets today, it often takes many years to see compounding breakaway moats.
This style of investing also has mature frameworks that VCs love to pattern match against. Things like selling pickaxes during a gold rush and transitioning industries from asset-heavy to asset-light while capturing the same value as asset-heavy approaches. Investors are in theory isolating variables and minimizing the need to be correct on various sequentially tied together futures, which is very hard.
And then there are incentives, which means this is a wildly profitable strategy on paper.
Investors stay one step ahead of the capital stack that looks to the prior stage as the “known universe” for investing and capture mark ups in years 1-4 as they raise new funds in years 3-5, ad infinitum, with either a win, a new story to tell (I know fund 3 blew up but this NewCo that got marked up in fund 4 is a monster) or with a bunch of fees such that the un-levering/churn of their LP base means they go from making tens of millions of dollars per year down to single digit millions, over a 20 year timespan.2Or the best of both worlds, you sell part of the management company because fee income streams are more durable than any vertical SaaS co that has ever existed.
This works until it doesn’t, of course, but by then the only thing in a worse spot is potentially an investor’s legacy (and maybe LPs’ wasted opportunity cost).
AI and Forecasting
As AI has continued to progress, we have begun to have many ground truth and first-order effects work their way into the market that has caused large amounts of “conviction”.3Of course these grounds truths today started as some form of first- and second-order forecasts yesterday.
Alongside this has come a level of consensus wisdom that has proliferated through both sides of the market (builders and investors). This started with people directly associating model parameter size with quality, which caused many to dig in a layer deeper and progress to understanding the most notable concept of Scaling Laws. Scaling Laws becoming a common nomenclature amongst investors from 2022 to 2024 has now led to people looking at this concept as a global truth and applying it to other adjacencies…like robotics.
This has led to the fastest Schelling point resulting from “second-order forecasting” I have ever seen in venture capital.
How did this happen?
Again, using the prior forecasting framework and applying it to AI is instructive to understand what has happened within the adjacency of robotics and the explicit bet that is driving investors’ blind optimism.
It is important to remember that forecasting has various degrees of consensus built in and this is a non-exhaustive list of the most obvious and most consensus forms of forecasting, shown by both the implicit and explicit bets that investors and founders are making on AI progression.
0 Order-Forecasts (Ground Truth)
- GPT-4 is a highly performant LLM and multimodality is working as well.
- Compute and data is needed to scale LLM performance (we can debate whether scaling laws will hold etc. but just take this as ground truth)
With these ground truths in place we then can look at some various forecasts and the resulting behaviors based on how people view them.
1st-Order Forecasts
What is the chance that somebody can unseat OpenAI as the leading frontier model developer?
This caused a bunch of people to deploy money into both OpenAI and also to all the other new labs like Anthropic, Mistral, etc. albeit with somewhat different theses for each. People were betting on everything across commoditization curves, the transfer of “secrets” being the core IP in AI labs (not the actual technology), an uptake of sovereign models, and much more.
Who are clearest immediate beneficiaries of AI model performance increases?
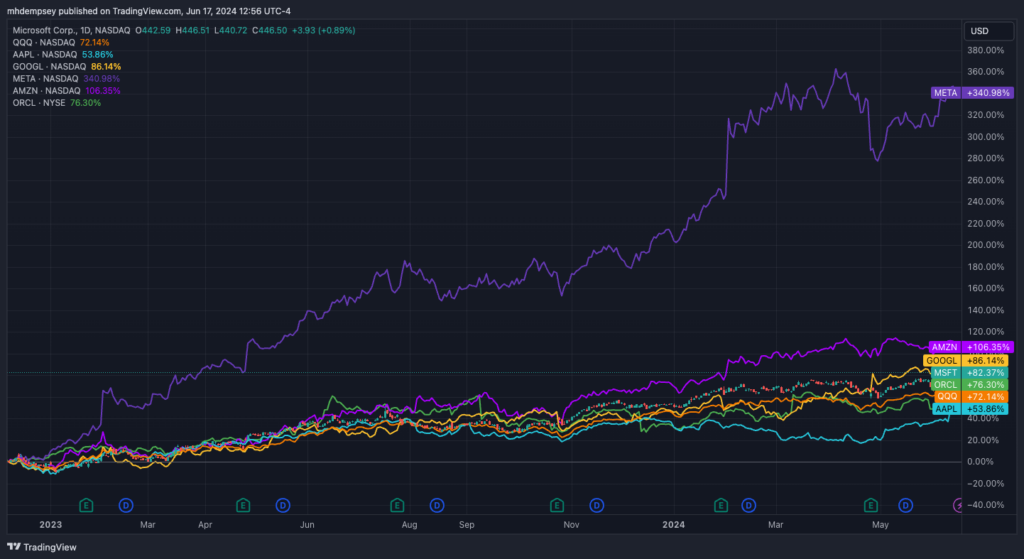
This caused people to long hyperscalers, some of which took longer than others for people to build conviction on. Meta was a clear beneficiary as they had products that could generate cash flow at higher efficiency from AI broadly, they benefitted from the commoditization of models, and they had accumulated large-scale compute.
Alphabet was a slower beneficiary as people were overly focused on the lost lead in AI despite largely being the most competent AI organization of the past decade+.
Microsoft was the most obvious trade with both distribution as well as a theoretical stranglehold on OpenAI (the Apple news maybe sheds some doubt on this).
Meanwhile, narratively Apple was moving too slow and was done and then everyone seemed to remember that they have insane local compute penetration/distribution and the stock went up $300B+ on some AI demos courtesy of Apple AI + OpenAI.
And now with companies like Adobe guiding up at earnings (and seeing material stock price appreciation because of it) investors are starting to look at a rotation of value creation from hyperscalers to more vertically-focused companies as the dust begins to settle on understanding a small amount on enterprise advantage accumulation of AI after initially worrying about broad-based destruction.
What industries can existing AI models serve well?
This is perhaps the most important first-order forecast for VCs and founders. This has led to what I would euphemistically call an aggressive funding of low-hanging fruit within the AI ecosystem and a dynamic where you have a large number of companies targeting the high-level use-cases of legal, research, accounting, marketing, and more.4Yes there are different products within these verticals as well
Who makes GPUs and will the supply/demand imbalance persist?
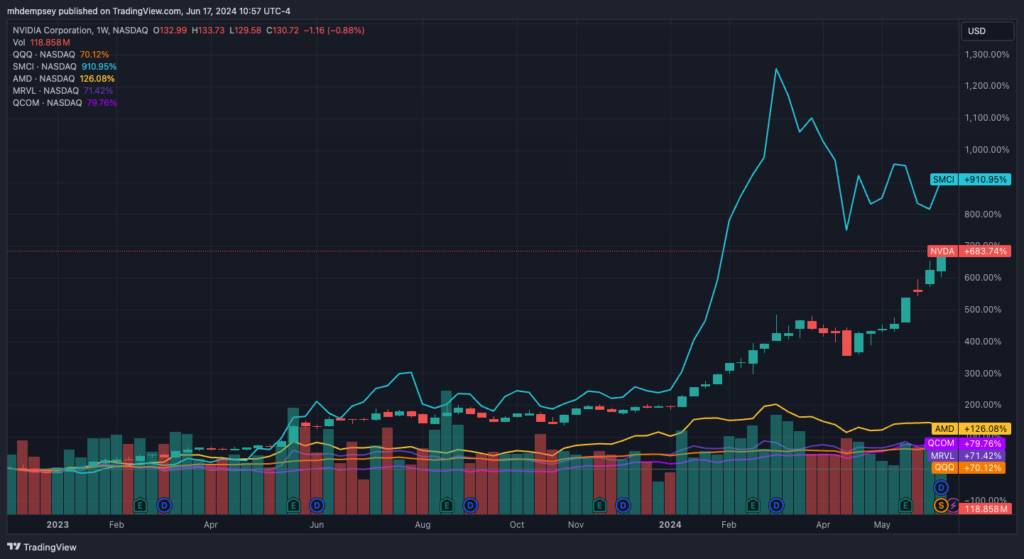
This caused people to long Nvidia and then adjacencies like SuperMicro (SMCI) and many others with haste while speculating on who was offside and would transfer market cap from incumbent to upstart (more on this later).
2nd-Order Forecasts
As we work our way through these we start to understand the derivatives of these first order implications. This is why I even started to write this schizophrenic post.
Will new types of infrastructure and dev tools need to be built to enable the AI application layer companies?
To our prior point on “pickaxes”, this is the most common derivative and easiest framework to observe within venture. Within months of ChatGPT we had companies pop up that noticed deficiencies of GPT-3/3.5 and tried to duct tape them up with narrow wedges and the hope to further expand into either more horizontal developer platforms or create new types of tools that are “AI specific”.
The world exploded about chain-of-thought, prompt engineering and eventually progressed into things like compute optimization, dataset evaluation, and more. This was “seeing the present very clearly” with seemingly minimal view of the future, as models improved performance and collapsed some of these “tools” into the native model UI/API call.
Admittedly, this is a very profitable way to invest if done surgically or in massive volume, as new platforms often necessitate new paradigms of infra and dev tooling. That said, it often feels the early movers in these types of moments are not advantaged unless they build a very large critical mass of developers and can pivot quickly, which often for some reason doesn’t happen as easily with heavily funded teams.5This is anecdotal.
Instead what has happened in AI is the earliest platforms, like Databricks, have shown very strong proficiency in understanding how to bolt-on interesting tools like MosaicML and LilacML, among others, via M&A.
There is perhaps another conversation to be had surrounding how long these moments necessitate highly offensive acquisitions (versus defensive). Both Mosaic and Tabular were venture-scale outcomes, but as we’ll talk about later, after a first-run of highly-valuable M&A that is often also driven by the acquirers stock price appreciation, many over-rotate on multiples for businesses in a given emerging category and are instead left with an expectation of persistent premium acquisitions and a reality of rationale M&A prices, as we have seen with early waves of M&A in mobile, cloud, AVs, and more.
Will the supply/demand imbalance of GPUs persist?
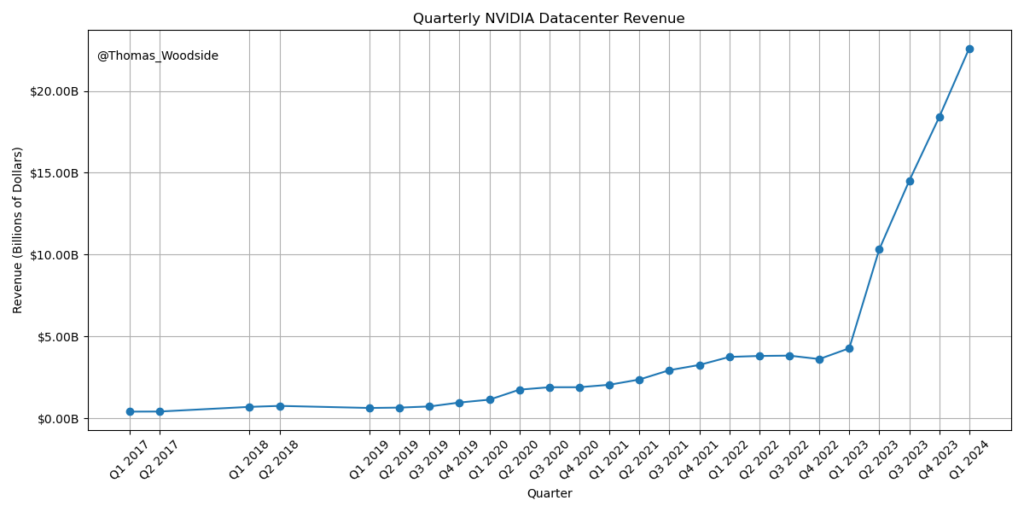
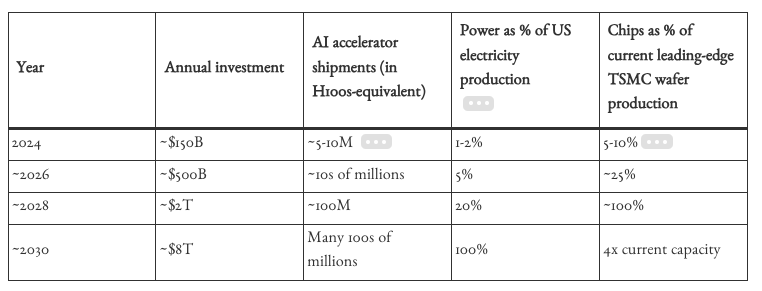
This forecast has many currently thinking about whether to be long or short NVDA at this current market cap (~$3.2T) as well as to back novel compute approaches focused on solving bottlenecks or having higher performance/efficiency for AI-specific problems.
What’s interesting about this is that there was a lot of money deployed against this thesis in the prior AI cycle because many believed deep learning would need custom compute that Nvidia wasn’t going to own the vast majority of market share wise.
Investors lost a lot of money but one could argue the viewpoint of “general GPUs won’t serve AI performance” didn’t work but morphed into “specialized compute can amplify AI performance” or “the world just wants more fucking compute”6These are same same but different) but think Mythic, Graphcore, Cerebras, Tenstorrent, Groq, and others. The implicit bets within these investments that *didn’t* work was likely also that the scale of AI performance and investment we are seeing today was going to take a bit longer to inflect than 2023, thus allowing the actual production scale to be achieved by some of these companies. It’s almost a shame that ZIRP and AI didn’t happen at the *exact* same time, otherwise likely every single compute manufacturer would have collected nine-figure rounds at a growth round instead of dying.7To be clear, we just have a different ZIRP now which is AI.
This forecast also has investors across different asset classes speculating on overbuilding or under-building of data centers as well as various other physical “pickaxe” style investments surrounding Compute As The New Oil and the expansion of clusters. Friends at Hedge funds, PE firms, real estate firms, and across various governments had never cared about my view on this question (or maybe anything related to AI?) up until about 12 months ago.
Will scaling laws hold for performance in other categories?
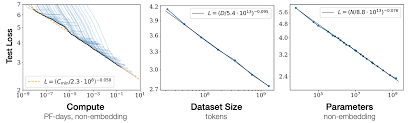
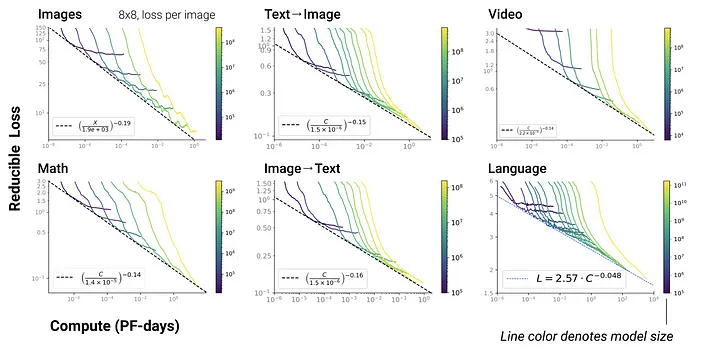

Language Models a Mirage?
This is perhaps the most important forecasting question in 2024 for venture capital (and the world) and is what has arguably led to a variety of “Foundation Model for x” companies.8Even when some of the datasets in the foundation models might just…end up in the frontier models?
If the problem of AI architecture selection is “solved” and locked into place9this is a HUGE if (transformers for AGI + perhaps some other System 2 depending on use-case for ASI) one can then think that increasing all types of AI model performance means following scaling laws and adding data + compute for a given number of modalities or use-cases.10This perceived breakthrough may be correct, however when the end product is not an API that is an aligned model that feeds a direct output as the ROI, but instead a resulting product in some form, this thinking can start to break down. I would argue this is one of the more poorly thought through dynamics within AI today.
This is what finally brings us to robotics and asking the question:
Will scaling laws hold for performance in robotics?
If you answer this question as “yes”, you enter into a third-order forecast of “will robotics be the most valuable category where scaling laws will hold?” alongside other questions such as data availability, synthetic data generation and its quality, and maybe you even get into the rabbit hole of energy and trillion dollar clusters.
If you answer the original question “no” then you may be one of the few people in AI who isn’t super bullish on robotics.
People can debate the modality (humanoid vs. not) but it’s hard to bet against consistent trend lines across nearly every other use-case. That said, we are not here to litigate whether this statement is true or not and instead I would recommend this great post on the topic.
On Robotics and Conviction
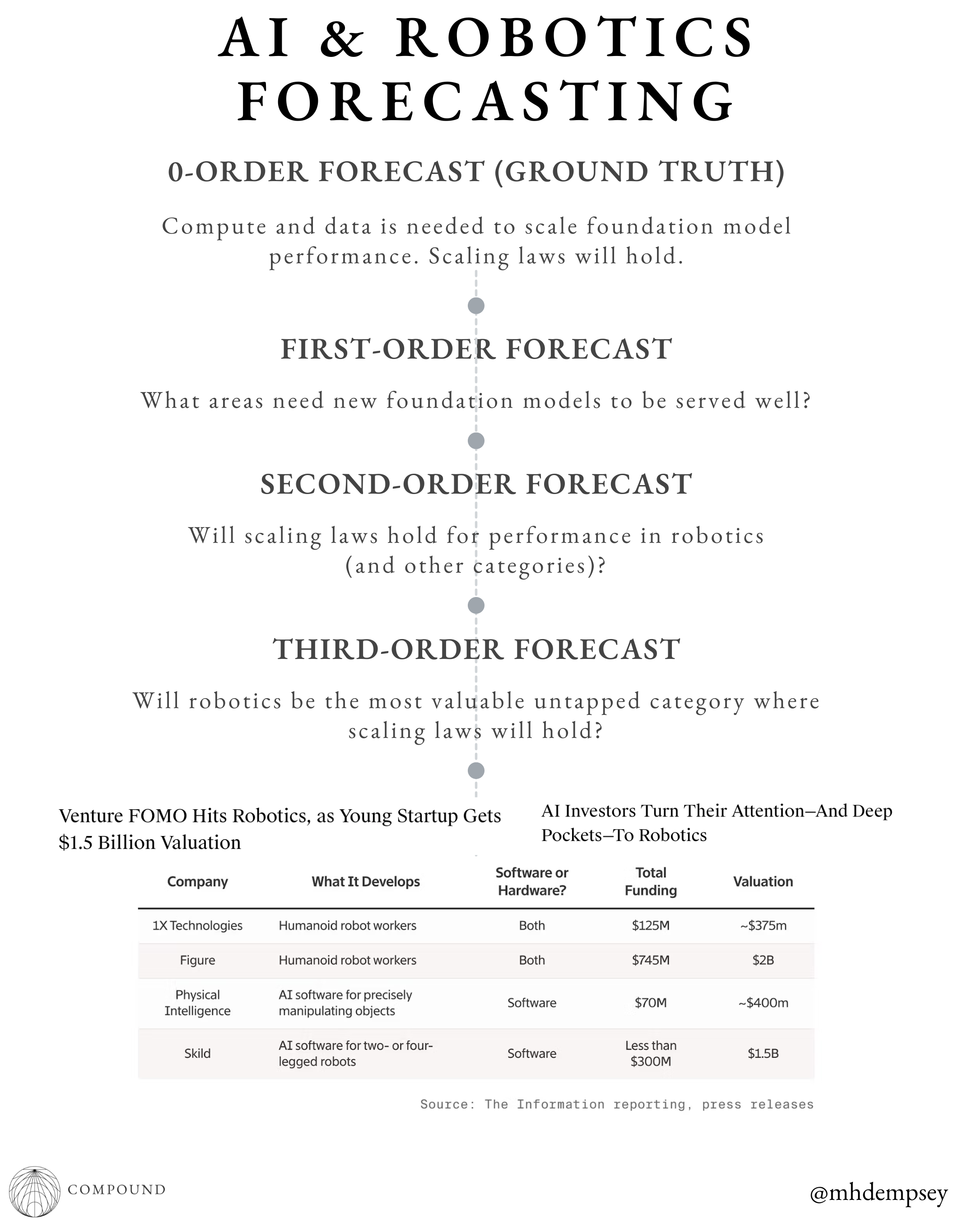
The reality of venture capital in 2024 is that funds are incentivized in some way to figure out where they can spot absolute upside11$5B+ outcomes are sorta all that matter for $1B+ funds as well as areas that can absorb the scale of capital that fit within the model of selling 10-30% of a business per round.
Robotics has been chosen as a prime candidate that satisfies the second-order effect of being a massive opportunity, as well as this framework of putting dollars to work,12and to be honest, all categories have been pushed into fitting in this framework, leading to areas that should be capital efficient ending up…not and even better there have been a very low number of startup competitors over the years in this space that have survived
Going back to my prior point, when an inflection point happens typically the processing of second- and third-order effects takes time both from an educational standpoint (How do i reason to being high conviction on these futures first-hand?) as well as a company creation standpoint (a subset of founders need time to gain confidence in the right company to build that they feel can meaningfully disrupt or outperform the incumbents that they often work for).
There however are times where these inflection points send reverberations throughout tight-knit, highly socialized industries, that the act of company creation is materially accelerated.
This happens for two main reasons on each side of the table of Capital and Talent.
Talent Dispersion post inflection points
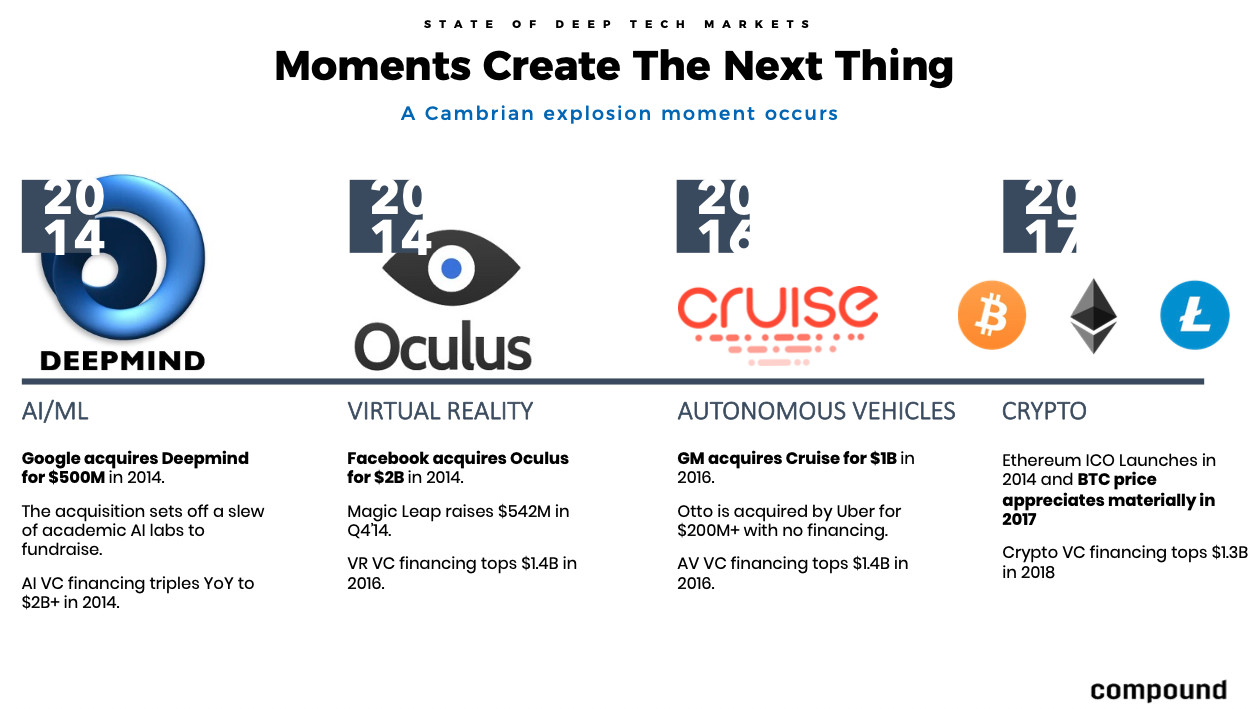
Talent agglomeration/dispersion happens because humans are humans, and thus specialized talent often moves far more in concert than people appreciate in emerging categories.
When talent starts to disperse it usually is after an event of sorts and in startups it can happen when a small subset of people are seen to have made a ton of money from a Cambrian Explosion moment for an industry. Rarely are these people viewed as the “elite” in the industry or the OGs, but rather some orthogonal group that rose from outside of the incumbents (because hyperscalers have been great at accumulating on-paper elite talent).
In Autonomous Vehicles we saw this with the Cruise acquisition causing an acceleration of talent dispersion outside of companies like Tesla, 510 Systems/Waymo, and even eventually Cruise itself once the first equity cliff was reached post-acquisition.
The AV talent diaspora started a variety of companies with varying degrees of novelty or real advantages but a directional view that either:
a) Incumbents were vulnerable to disruption because well, if Cruise could do it so could another startup
or
b) There would be an entire economy to emerge around autonomous vehicles (delivery robots, trucking, etc.) or other tools (simulation, pedestrian understanding, etc.) I wrote about this dynamic back in 2016 and presented 3 possible futures including:
3) Never deploy — This option is why most talented engineers sitting at larger automotive companies are at least thinking of starting a company, raising a “pre-seed” round of at least $500K-$1M, and riding the hype cycle perfectly. And I don’t blame them.
You read the insane article that states that AV acquisitions are happening at $10M/engineer and you run some math on how much money that is for you and your team. You raise that pre-seed, build something compelling enough, raise a more formal seed round to scale it to different types of production vehicles, driving scenarios, location types, etc. and then you pray you never have to go to market because options 1 and 2 sound like hell. So instead you find a nice OEM, a larger more well-funded team, or a tier 1 supplier to fit the bill for the next few years of R&D that it will take to get to market, in the form of an early acquisition.
Your VCs are probably a little pissed, but who cares, because every VC is also sitting there telling themselves that these AV bets are downside protected due to these exact scenarios. Until they’re not.
In AI we arguably saw the incubation period of this after the Deepmind acquisition in 2014. Shortly thereafter, new AI labs emerged until an AI winter of sorts hit in ~2017, eventually killing most except for that weird non-profit/capped profit/open-source/closed-source thing called OpenAI which started in late 2015.13There maybe is a separate lesson here that we should all be tapping into philanthropy money first for large-scale ambitious things with no clear cut financing market and then flipping the orgs, but we’ll see.
From there it was a slow build until the emergence of GPT-2 in 2019 and GPT-3 (2020) led to a new exodus of talent from the major AI labs as they saw both a possibility for commercialization and as importantly, a willingness for someone to fit the bill for all of the compute an independent entity would need.14Maybe some ethical considerations as well.
Capital had arrived.
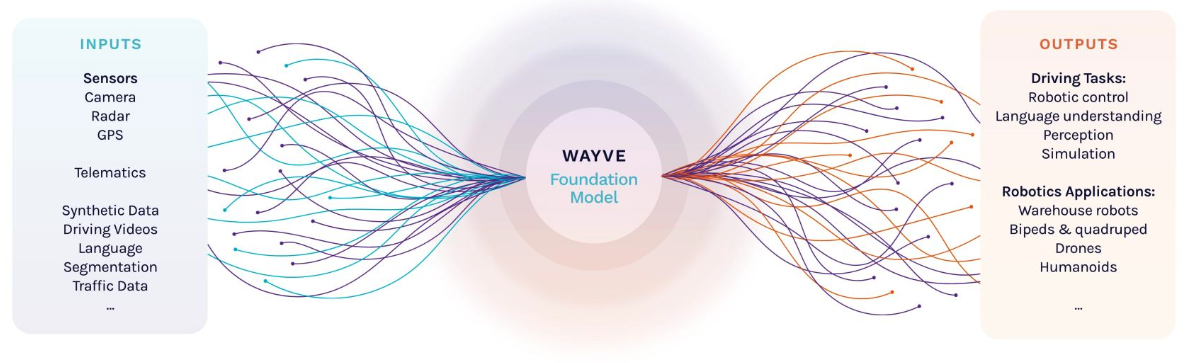
With robotics, we have now seen a similar kickstarting as top labs like Deepmind/GoogleAI alongside companies like Wayve15A Compound portfolio company have shown breakthroughs in embodied intelligence that point to the possibility to transferring this to other domains, leading to the incumbents (DeepGoog, Tesla, etc.) to begin losing talent.
And to enable this, once again, capital has arrived.
Capital Acceleration & DEployment
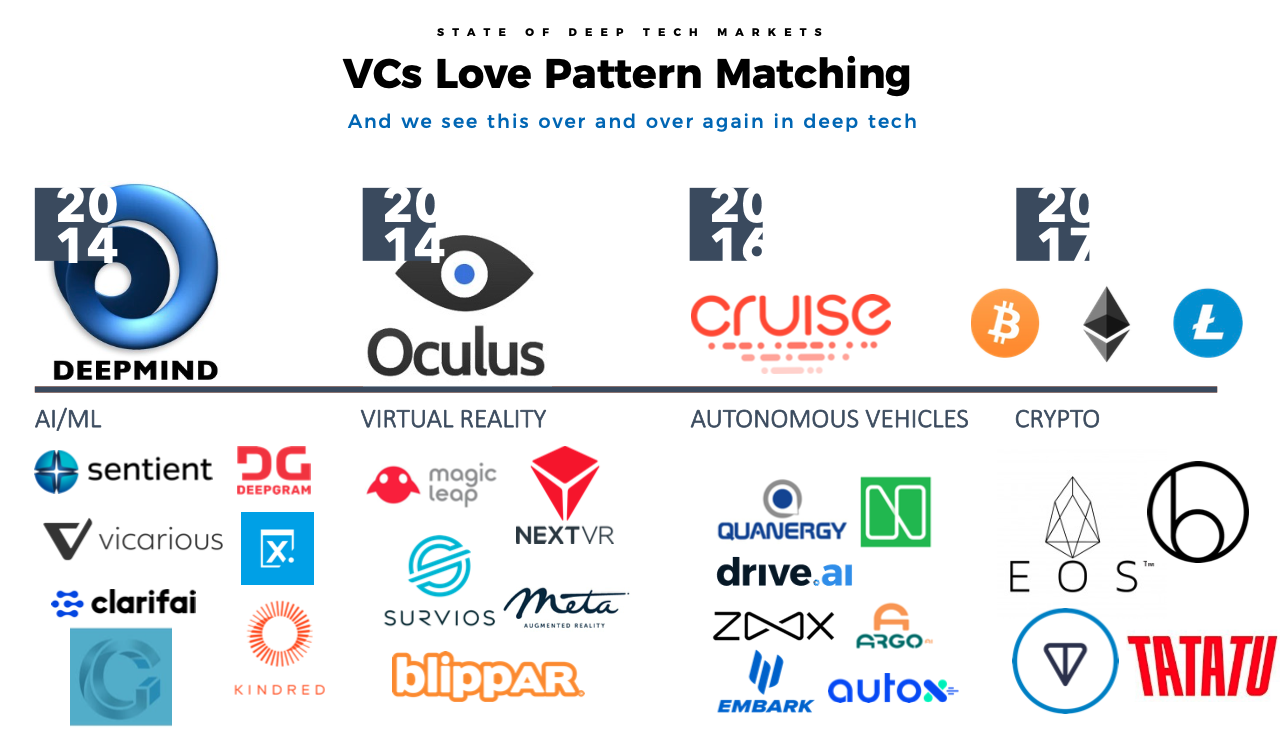
Capital acceleration in new markets and emerging adjacencies happens from similar principles as above.
A moment happens that gives conviction to investors that they can feasibly make money, often catching most off-guard and underexposed to the theme. This compounds as GPs/LPs start asking when more money will be deployed to fix the underexposed positioning.
Naturally, investors start their search at the “elite” places for talent, often large industry labs and academia (I wrote about this dynamic in-depth previously). As startups have industrialized, two things have happened at this stage that has been enabled by capital.
Playing Venture Games
The top talent now understands their market signal and use it to capture large sums of capital to go after a broader “area” with a looser idea of product/GTM than many boomer investors expect.16I say this tongue in cheek They observe the supply/demand imbalance of top talent, and thus raise enough money up front to rip out a large chunk of their current team/lab or a collection of labs under the (correct) thesis that a focused startup can make more progress than a constrained R&D group in a large company or institution.17This happens at times for political reasons and other times for talent retention reasons (in academia) among others.
The earlier generations of this looked like a top professor being pulled by a few great students18Covariant with Pieter Abbeel is a good example of this, then progressed to larger groups led by top executives, like Nuro/Aurora or Anthropic, each splitting off with very large fundraises and collections of talent aimed at doing what the incumbent was doing but better or slightly adjacent (in Nuro’s case).19You also had some weird ones like Ike who split from Nuro but licensed the tech to do trucking.
In AI, it’s even more clear this pillaging of academia will continue to happen as maybe for the first time in history, the dollars in large not-for-profit institutions aren’t properly allocated to empower the research/science. Put simply, universities and research orgs aren’t buying enough GPUs to push forward experiments that require scale of any kind.
Fragmentation, Large Pools of Capital, and Intense Competition.
Venture investors can’t help themselves but to try to kingmake companies with capital. Eventually, the foie-grasification of startups happens because capitalism and fear of competition, a self-fulfilling prophecy that leads to a collapse of multiple funding rounds into one.
I am of the belief that companies should almost always do the maximally ambitious (and value capturing) thing over a 10-15 year time horizon, while compounding learnings from smaller milestones along the way. A potential discrepancy between myself versus the market is that others believe instead of sequencing to a future you believe in, you shouldn’t even waste time focusing on the smaller things and instead should always go from 0 to a general purpose, fully autonomous, humanoid robot, and nothing in the middle matters.
This dynamic is viewed as dominant or table stakes by both founders and VCs because of “competition”, however I think this often breeds poor discipline and strategic thinking on figuring out what is most important for a company to get to their core/hero milestone before raising large sums of money to push on those advantages. In addition, it makes companies far less malleable to exogenous shifts than they anticipate or realize.
We have now seen this financing dynamic play out for very ambitious startups started in the past ~24 months like Physical Intelligence, Skilld, Bot.co, Mentee, 1x, Sanctuary, and many others.
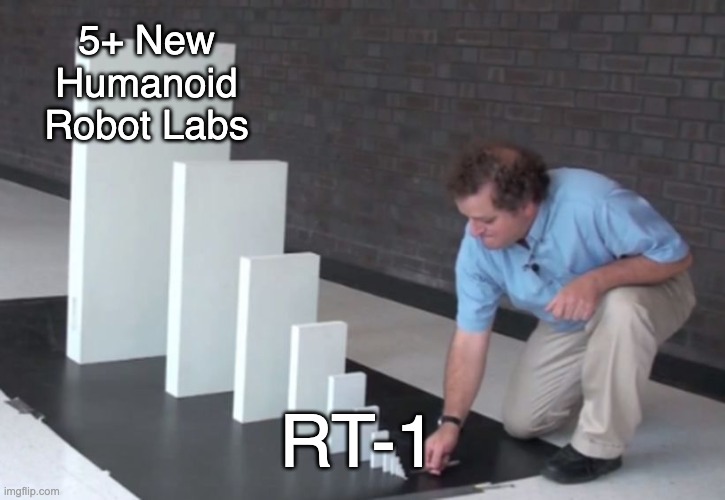
A domino effect of research breakthroughs → talent uneasiness (am i wasting my life at insert lab?) → cap table formation (we should start a company) → capital accumulation ($50M+ seed round).
I am often reminded by (and repeat) Kwok’s wisdom that “at some point in the past decade people in tech realized if they ask for $1B, they might end up with $200M, and if they ask for $3M, they might end up with $0.”
Robotics as a viewpoint of Innovation Capital
Perhaps in some ways the intense speed at which the robotics narrative caught hold within both the technical as well as venture ecosystems is a symptom of where technology is today.
There are very few stones left unturned, there are large amounts of money chasing pools of ideas that rotate narratively quite quickly, and there is a willingness to maximally extrapolate single datapoints into long-term durable ones.
Foundation Models in particular have a certain gravity to them that is undeniable at a time where scaling laws cannot be disproven. We have been told by the smartest minds and best AI fundraisers in the world that all you need to do is pile a bunch of data, GPUs, and smart people in a room, learn some secret sauce (or poach someone that already did it at a competing lab) on how to properly train these models, and then you get a god-like machine for *any* use-case.
All anyone asks is how much will it cost to build the machine, how do you get the data and maintain it, and *maybe* what makes you special versus everyone else?
If we look back at 2020/2021, there was high conviction in a variety of narratives within tech that led to historic levels of capital being raised and deployed. E-Comm scaling, enterprise cloud penetration, financial institution incumbent disruption, emerging markets like LATAM and the Middle East maturing, deep tech companies being appreciated for their 2025E revenue, and on and on it went.
I’ve often thought about what lessons would last from the fervor of 2021 and how long it would take people to forget, and as I wrote these prior two paragraphs, I realized I had written and talked about this dynamic before in 2022 as it related to software companies broadly. We all saw Software Eat The World and then all bet it would continue to eat everything else and bid things up to extreme levels only for the classic loss ratios and valuation compressions to occur as the 2021 SaaS et al bubble popped.
I sometimes wonder what will happen if scaling laws don’t hold and if that will look similar to what revenue churn, slowed growth, and higher interest rates did to many parts of tech. I also sometimes wonder if scaling laws perfectly hold and if that will look similar to what commoditization curves in many other areas have looked like for first movers and their value capture.
The nice part about capitalism is either way we’re going to light a ton of money on fire finding out.
Again, this essay is not about declaring what is correct or incorrect but rather observing how these phenomena unfold. A core takeaway from the robotics zeitgeist rate of progression/consensus is that alpha in startups may no longer lie in merely seeing the present and near future clearly.
The present is often overrun by consensus and capital, leading to inflated valuations, aggressive competition, and diminished returns. Instead, the future of company and investing advantages might lie in understanding the nuanced and complex interplay of technological advancements and market dynamics, and in anticipating the deeper, less obvious second and third-order effects.
The current fervor around robotics, particularly humanoid robots, exemplifies this shift. Investors are betting on the most generalizable approaches, driven by the idea that what works in AI will seamlessly translate to robotics. This simplified forecasting could be a testament to the improved speculative ability of modern investors to let go of the meme of “consensus vs. contrarian” and bet on all trend lines progressing. Or, it could be a sign of overly simplistic thinking at a time where we chase every shiny object that pops up in front of us without stopping to litigate and add nuance.
The answer likely lies somewhere in the middle, but either way, we continue to push further out onto the risk curve in search of outlier outcomes.
Recent Comments